Ship classification in high-resolution (HR) synthetic aperture radar (SAR) images is an important and challenging task. The deep learning methods rely heavily on massive labeled data, which is not practical for SAR applications due to the lack of feature consistency under different sensors, angle of view, and scenarios, while the statistical methods are vulnerable to noise, image quality, and radiometric calibration and have poor robustness. To overcome these problems, a more robust superstructure scattering feature, named scattering bright line feature, is proposed to realize the convenient classification of ships in engineering applications. Based on this, a decision tree classifier for three types of merchant ships is developed. Four types of HR SAR slices are used to verify the validity of the new feature by both the decision tree and support vector machine classifiers, and the results show that the proposed feature has good classification performance in merchant ships with an overall accuracy higher than 80%. |
ACCESS THE FULL ARTICLE
No SPIE Account? Create one
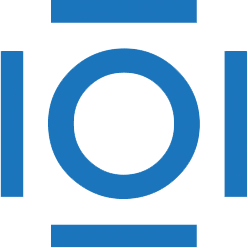
CITATIONS
Cited by 2 scholarly publications.
Synthetic aperture radar
Scattering
Space based lasers
Feature extraction
Image classification
Signal to noise ratio
Image resolution