With the development of technology, it is becoming easier and easier for people to generate tampered images that are indistinguishable from the human eye, and the malicious use of these photos in news, academic papers, and criminal crimes has brought great harm to society. This paper proposes a deep convolutional neural network-based image forgery localization method to uncover the subtle differences between doctored images and real images. Specifically, the network achieves tampering localization through a three-stage enhancement scheme. First, the dilated convolution in the deep layer of the network is used to keep the feature map resolution constant, and the number of shallow convolutions is decreased to reduce the perceptual field, so the network focuses on local regions. Second, the feature enhancement module is used to fuse shallow features with deep features to effectively filter content information and highlight tampering features, making full use of local and global information to improve the generalization ability. Finally, the attention enhancement module reweights the convolutional feature maps in terms of channels and locations, respectively, to highlight the information regions around the forgery boundaries, thus guiding the network to capture more intrinsic features for image forgery. Extensive experimental results on several public datasets show that this method outperforms other state-of-the-art methods in image forgery localization. |
ACCESS THE FULL ARTICLE
No SPIE Account? Create one
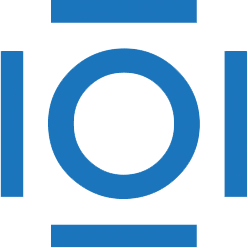
CITATIONS
Cited by 2 scholarly publications.
Image enhancement
Convolution
Feature extraction
Education and training
Image forensics
Quantization
Semantics