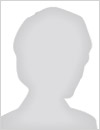
Thrasyvoulos N. Pappas received the S.B., S.M., and Ph.D. degrees in
electrical engineering and computer science from the Massachusetts
Institute of Technology, Cambridge, MA, in 1979, 1982, and 1987,
respectively. From 1987 until 1999, he was a Member of the Technical
Staff at Bell Laboratories, Murray Hill, NJ. In 1999, he joined the
Department of Electrical and Computer Engineering (now EECS) at
Northwestern University as an associate professor. His research
interests are in image and multidimensional signal processing, and in
particular, image and video quality and compression, image and video
analysis, content-based retrieval, video transmission over
packet-lossy networks, perceptual models for image processing,
model-based halftoning, tactile devices, processing, and perception,
and multimodal signal processing.
Dr. Pappas is a Fellow of IEEE and a Fellow of SPIE.
He is Editor-in-Chief of the IEEE Transactions on Image Processing (2010-12).
Dr. Pappas has served as an elected member of the Board of Governors
of the Signal Processing Society of IEEE (2004-7), chair of the
IEEE Image and Multidimensional Signal Processing Technical Committee
(2002-3), and associate editor of the IEEE Transactions on Image
Processing (1996-2003), the IEEE Signal Processing Magazine
(2006-8), the SPIE/IS&T Journal of Eletronic Imaging (2007-9), and
the Foundations and Trends in Signal Processing Journal (2006-9).
He has also served as technical program co-chair of ICIP-09, ICIP-01,
and the Symposium on Information Processing in Sensor Networks (IPSN-04).
Since 1997 he has been co-chair of the SPIE/IS&T Conference on Human
Vision and Electronic Imaging. He has also served as co-chair of the 2005
SPIE/IS&T Electronic Imaging Symposium.
electrical engineering and computer science from the Massachusetts
Institute of Technology, Cambridge, MA, in 1979, 1982, and 1987,
respectively. From 1987 until 1999, he was a Member of the Technical
Staff at Bell Laboratories, Murray Hill, NJ. In 1999, he joined the
Department of Electrical and Computer Engineering (now EECS) at
Northwestern University as an associate professor. His research
interests are in image and multidimensional signal processing, and in
particular, image and video quality and compression, image and video
analysis, content-based retrieval, video transmission over
packet-lossy networks, perceptual models for image processing,
model-based halftoning, tactile devices, processing, and perception,
and multimodal signal processing.
Dr. Pappas is a Fellow of IEEE and a Fellow of SPIE.
He is Editor-in-Chief of the IEEE Transactions on Image Processing (2010-12).
Dr. Pappas has served as an elected member of the Board of Governors
of the Signal Processing Society of IEEE (2004-7), chair of the
IEEE Image and Multidimensional Signal Processing Technical Committee
(2002-3), and associate editor of the IEEE Transactions on Image
Processing (1996-2003), the IEEE Signal Processing Magazine
(2006-8), the SPIE/IS&T Journal of Eletronic Imaging (2007-9), and
the Foundations and Trends in Signal Processing Journal (2006-9).
He has also served as technical program co-chair of ICIP-09, ICIP-01,
and the Symposium on Information Processing in Sensor Networks (IPSN-04).
Since 1997 he has been co-chair of the SPIE/IS&T Conference on Human
Vision and Electronic Imaging. He has also served as co-chair of the 2005
SPIE/IS&T Electronic Imaging Symposium.
This will count as one of your downloads.
You will have access to both the presentation and article (if available).
We also present new testing procedures for objective texture similarity metrics. We identify three operating domains for evaluating the performance of such similarity metrics: the top of the similarity scale, where a monotonic relationship between metric values and subjective scores is desired; the ability to distinguish between perceptually similar and dissimilar textures; and the ability to retrieve "identical" textures. Each domain has different performance goals and requires different testing procedures. Experimental results demonstrate both the performance of the proposed metrics and the effectiveness of the proposed subjective testing procedures.
This will count as one of your downloads.
You will have access to both the presentation and article (if available).
We will examine objective criteria for the evaluation of image quality that are based on models of visual perception. Our primary emphasis will be on image fidelity, i.e., how close an image is to a given original or reference image, but we will broaden the scope of image fidelity to include structural equivalence. We will also discuss no-reference and limited-reference metrics. We will examine a variety of applications with special emphasis on image and video compression. We will examine near-threshold perceptual metrics, which explicitly account for human visual system (HVS) sensitivity to noise by estimating thresholds above which the distortion is just-noticeable, and supra-threshold metrics, which attempt to quantify visible distortions encountered in high compression applications or when there are losses due to channel conditions. We will also consider metrics for structural equivalence, whereby the original and the distorted image have visible differences but both look natural and are of equally high visual quality. We will also take a close look at procedures for evaluating the performance of quality metrics, including database design, models for generating realistic distortions for various applications, and subjective procedures for metric development and testing. Throughout the course we will discuss both the state of the art and directions for future research.
Course topics include:
• Applications: Image and video compression, restoration, retrieval, graphics, etc.
• Human visual system review
• Near-threshold and supra-threshold perceptual quality metrics
• Structural similarity metrics
• Perceptual metrics for texture analysis and compression – structural texture similarity metrics
• No-reference and limited-reference metrics
• Models for generating realistic distortions for different applications
• Design of databases and subjective procedures for metric development and testing
• Metric performance comparisons, selection, and general use and abuse
• Embedded metric performance, e.g., for rate-distortion optimized compression or restoration
• Metrics for specific distortions, e.g., blocking and blurring, and for specific attributes, e.g., contrast, roughness, and glossiness
• Multimodal applications
View contact details
No SPIE Account? Create one