|
ACCESS THE FULL ARTICLE
No SPIE Account? Create one
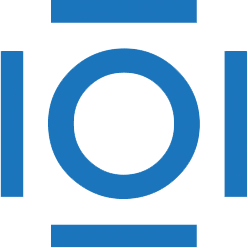
CITATIONS
Cited by 30 scholarly publications and 6 patents.
Computed tomography
Heart
Chest
Convolutional neural networks
Arteries
Computer aided diagnosis and therapy
Artificial neural networks