|
ACCESS THE FULL ARTICLE
No SPIE Account? Create one
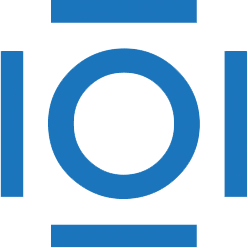
CITATIONS
Cited by 1 scholarly publication.
Feature extraction
Pathology
Breast cancer
Data modeling
Image classification
Convolutional neural networks
Image analysis