|
ACCESS THE FULL ARTICLE
No SPIE Account? Create one
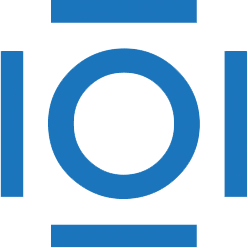
CITATIONS
Cited by 2 scholarly publications.
Breast
Image segmentation
Magnetic resonance imaging
Breast cancer
Edge detection
Imaging systems
Tissues