|
ACCESS THE FULL ARTICLE
No SPIE Account? Create one
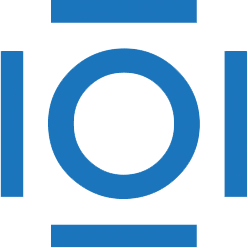
CITATIONS
Cited by 1 scholarly publication.
Magnetic resonance imaging
Image analysis
Nonuniformity corrections
Tissues
Gallium nitride
Image segmentation
3D image processing