|
ACCESS THE FULL ARTICLE
No SPIE Account? Create one
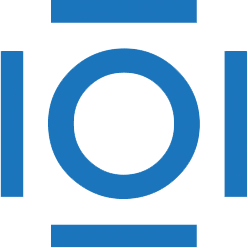
CITATIONS
Cited by 2 scholarly publications.
Remote sensing
RGB color model
Image quality
Clouds
Data modeling
Image processing
Neural networks