|
ACCESS THE FULL ARTICLE
No SPIE Account? Create one
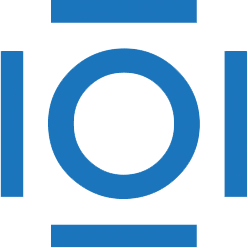
CITATIONS
Cited by 4 scholarly publications.
Image segmentation
Vegetation
Principal component analysis
Satellite imaging
Earth observing sensors
Computer programming
Near infrared