|
ACCESS THE FULL ARTICLE
No SPIE Account? Create one
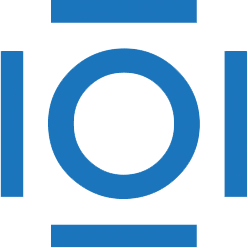
CITATIONS
Cited by 3 scholarly publications.
Chest imaging
Data modeling
Convolutional neural networks
Performance modeling
Visualization
Lung
Statistical modeling