|
ACCESS THE FULL ARTICLE
No SPIE Account? Create one
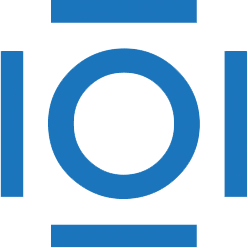
CITATIONS
Cited by 2 scholarly publications.
Neural networks
Convolutional neural networks
Matrices
Neurons
Machine learning
Principal component analysis
Stochastic processes