|
ACCESS THE FULL ARTICLE
No SPIE Account? Create one
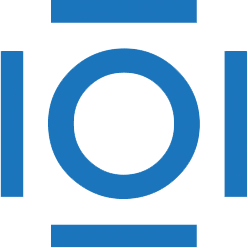
CITATIONS
Cited by 4 scholarly publications.
Image segmentation
Breast
Digital mammography
Breast cancer
Tissues
Convolutional neural networks
Data modeling