|
ACCESS THE FULL ARTICLE
No SPIE Account? Create one
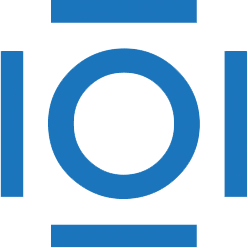
CITATIONS
Cited by 2 scholarly publications.
Mahalanobis distance
Neural networks
Artificial neural networks
Image classification
FDA class I medical device development
Automatic target recognition
Classification systems