|
ACCESS THE FULL ARTICLE
No SPIE Account? Create one
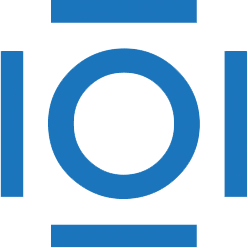
CITATIONS
Cited by 2 scholarly publications.
Neural networks
Object recognition
Acoustics
Signal to noise ratio
Submerged target modeling
Binary data
Electromagnetic simulation