|
ACCESS THE FULL ARTICLE
No SPIE Account? Create one
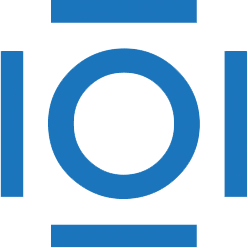
CITATIONS
Cited by 1 scholarly publication.
Autoregressive models
Image classification
Statistical analysis
Image segmentation
Statistical modeling
Image processing
Signal to noise ratio