|
ACCESS THE FULL ARTICLE
No SPIE Account? Create one
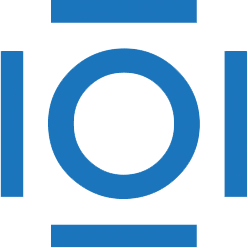
CITATIONS
Cited by 10 scholarly publications.
Mammography
Signal to noise ratio
Computer aided diagnosis and therapy
Image segmentation
Signal processing
Visibility
Feature extraction