|
ACCESS THE FULL ARTICLE
No SPIE Account? Create one
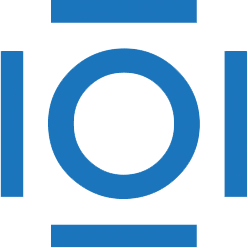
CITATIONS
Cited by 3 scholarly publications.
Neural networks
Fuzzy logic
Neurons
Fuzzy systems
Binary data
Mathematics
Genetic algorithms