|
ACCESS THE FULL ARTICLE
No SPIE Account? Create one
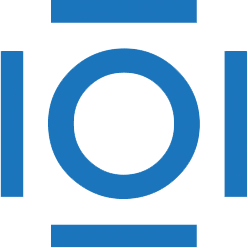
CITATIONS
Cited by 2 scholarly publications.
Image compression
Magnesium
Medical imaging
Principal component analysis
Feature extraction
Mathematical modeling
Image filtering