|
ACCESS THE FULL ARTICLE
No SPIE Account? Create one
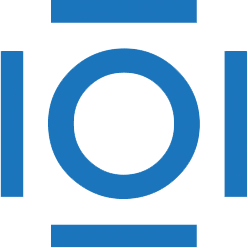
CITATIONS
Cited by 4 scholarly publications.
Signal to noise ratio
Optical spheres
Data modeling
Imaging systems
Statistical analysis
Point spread functions
Spherical lenses