|
ACCESS THE FULL ARTICLE
No SPIE Account? Create one
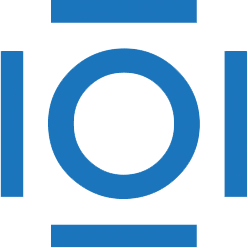
CITATIONS
Cited by 10 scholarly publications.
Neural networks
Signal to noise ratio
Data modeling
Signal processing
Systems modeling
Detection and tracking algorithms
Filtering (signal processing)