|
ACCESS THE FULL ARTICLE
No SPIE Account? Create one
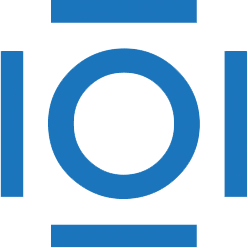
CITATIONS
Cited by 6 scholarly publications and 4 patents.
Picosecond phenomena
FT-IR spectroscopy
Machine learning
Resistance
Artificial neural networks
Neural networks
Spectroscopy