|
ACCESS THE FULL ARTICLE
No SPIE Account? Create one
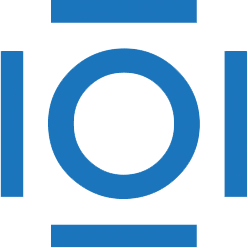
CITATIONS
Cited by 2 scholarly publications.
Wavelets
Global system for mobile communications
Denoising
Stochastic processes
Image processing
Algorithm development
Statistical modeling