|
ACCESS THE FULL ARTICLE
No SPIE Account? Create one
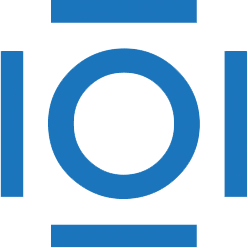
CITATIONS
Cited by 4 scholarly publications.
Detection and tracking algorithms
Diffusion
Target detection
Target recognition
Automatic target recognition
Filtering (signal processing)
Sensors