|
ACCESS THE FULL ARTICLE
No SPIE Account? Create one
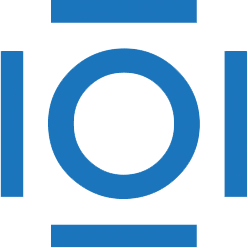
CITATIONS
Cited by 7 scholarly publications.
Object recognition
Detection and tracking algorithms
Feature extraction
Sensors
Classification systems
Machine learning
Simulation of CCA and DLA aggregates