|
ACCESS THE FULL ARTICLE
No SPIE Account? Create one
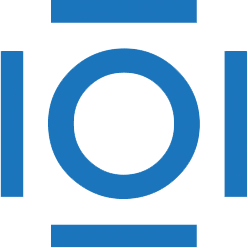
CITATIONS
Cited by 4 scholarly publications.
Wavelets
Diffusion tensor imaging
Denoising
Diffusion
Signal to noise ratio
Visualization
Brain