|
ACCESS THE FULL ARTICLE
No SPIE Account? Create one
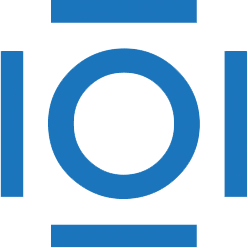
CITATIONS
Cited by 37 scholarly publications.
Image segmentation
Liver
Computed tomography
Magnetorheological finishing
Image classification
Tissues
Data modeling