|
ACCESS THE FULL ARTICLE
No SPIE Account? Create one
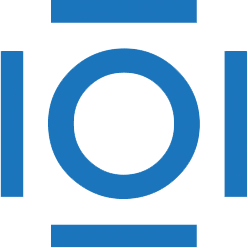
CITATIONS
Cited by 8 scholarly publications.
Image segmentation
Principal component analysis
Statistical analysis
Breast
Mammography
Tissues
Computer aided diagnosis and therapy