|
ACCESS THE FULL ARTICLE
No SPIE Account? Create one
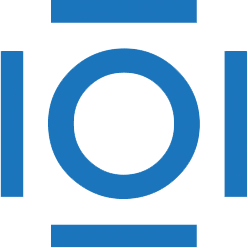
CITATIONS
Cited by 15 scholarly publications.
Vegetation
Image segmentation
Calibration
Detection and tracking algorithms
Signal to noise ratio
Cameras
Error analysis