|
ACCESS THE FULL ARTICLE
No SPIE Account? Create one
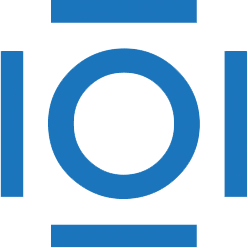
CITATIONS
Cited by 2 scholarly publications.
Atomic force microscopy
Principal component analysis
Image classification
Spatial frequencies
Edge detection
RGB color model
Feature extraction