|
ACCESS THE FULL ARTICLE
No SPIE Account? Create one
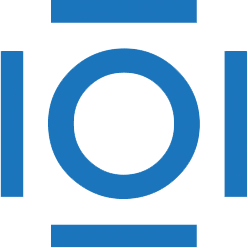
CITATIONS
Cited by 1 scholarly publication.
Infrared radiation
Infrared imaging
Infrared detectors
Thermal modeling
Computer programming
Infrared search and track
Statistical analysis