|
ACCESS THE FULL ARTICLE
No SPIE Account? Create one
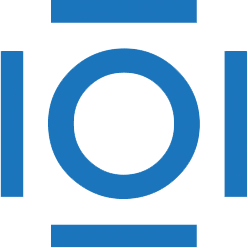
CITATIONS
Cited by 10 scholarly publications and 7 patents.
Image segmentation
3D modeling
3D image processing
Arteries
Image processing algorithms and systems
3D applications
Computed tomography