|
ACCESS THE FULL ARTICLE
No SPIE Account? Create one
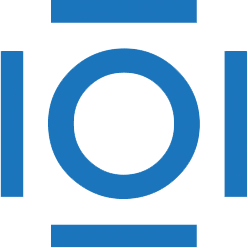
CITATIONS
Cited by 12 scholarly publications.
Data modeling
Electronic filtering
Sensors
Motion models
Mathematical modeling
Probability theory
Systems modeling