|
ACCESS THE FULL ARTICLE
No SPIE Account? Create one
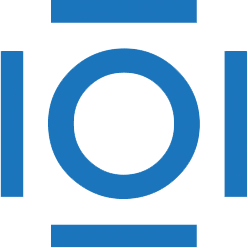
CITATIONS
Cited by 2 scholarly publications.
Soil science
Statistical analysis
Machine learning
Dielectrics
Data modeling
Biological research
Neural networks