|
ACCESS THE FULL ARTICLE
No SPIE Account? Create one
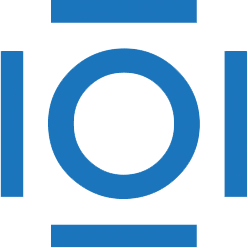
CITATIONS
Cited by 1 scholarly publication.
Particle filters
Navigation systems
Nonlinear filtering
Particles
Error analysis
Digital filtering
Inertial navigation systems