|
ACCESS THE FULL ARTICLE
No SPIE Account? Create one
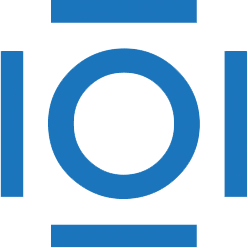
CITATIONS
Cited by 6 scholarly publications and 1 patent.
Machine learning
Visualization
Data modeling
Feature extraction
Image segmentation
Statistical analysis
Detection and tracking algorithms