|
ACCESS THE FULL ARTICLE
No SPIE Account? Create one
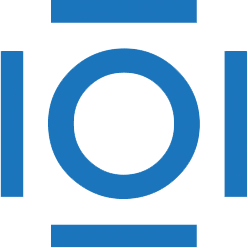
CITATIONS
Cited by 1 scholarly publication.
Video
Wavelets
Principal component analysis
Wavelet transforms
Bismuth
Feature extraction
Sensors