|
ACCESS THE FULL ARTICLE
No SPIE Account? Create one
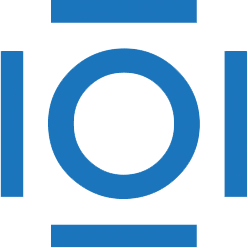
CITATIONS
Cited by 4 scholarly publications.
Defect detection
Diagnostics
Data modeling
Image quality
Feature extraction
Bandpass filters
Machine learning