|
ACCESS THE FULL ARTICLE
No SPIE Account? Create one
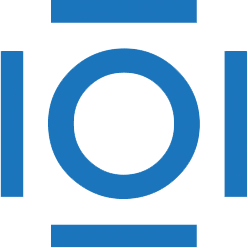
CITATIONS
Cited by 9 scholarly publications.
Associative arrays
Chemical species
Tomography
Image processing
Inverse problems
Principal component analysis
Reconstruction algorithms