|
ACCESS THE FULL ARTICLE
No SPIE Account? Create one
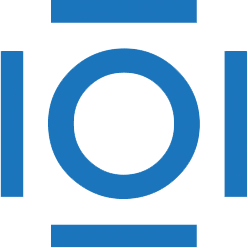
CITATIONS
Cited by 2 scholarly publications.
Data modeling
Neural networks
LIDAR
Neurons
Remote sensing
Statistical modeling
Calibration