|
ACCESS THE FULL ARTICLE
No SPIE Account? Create one
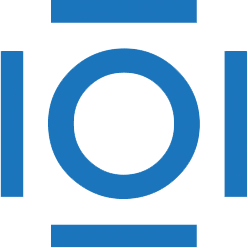
CITATIONS
Cited by 4 scholarly publications.
Data modeling
Detection and tracking algorithms
Hyperspectral imaging
Statistical modeling
Mathematical modeling
Image sensors
RGB color model