|
ACCESS THE FULL ARTICLE
No SPIE Account? Create one
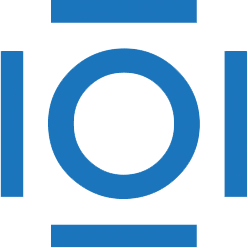
CITATIONS
Cited by 2 scholarly publications.
Statistical analysis
X-rays
Data modeling
Photon counting
Model-based design
Statistical modeling
Data acquisition