|
ACCESS THE FULL ARTICLE
No SPIE Account? Create one
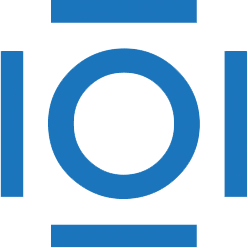
CITATIONS
Cited by 1 scholarly publication.
Sensors
Cameras
Data modeling
Sensor performance
Electroluminescent displays
Motion detection
Statistical modeling