|
ACCESS THE FULL ARTICLE
No SPIE Account? Create one
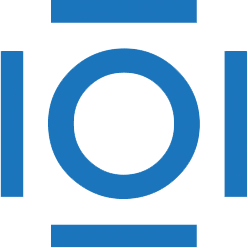
CITATIONS
Cited by 6 scholarly publications.
Reconstruction algorithms
CT reconstruction
X-ray imaging
X-rays
X-ray computed tomography
Denoising
Image restoration