|
ACCESS THE FULL ARTICLE
No SPIE Account? Create one
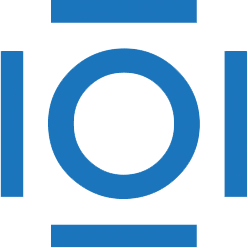
CITATIONS
Cited by 20 scholarly publications.
Binary data
Long wavelength infrared
Data modeling
Hyperspectral imaging
Gallium arsenide
Absorption
Feature selection