|
ACCESS THE FULL ARTICLE
No SPIE Account? Create one
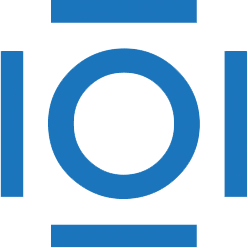
CITATIONS
Cited by 1 scholarly publication.
Vegetation
Radiative transfer
Biological research
Reflectivity
Agriculture
Inverse problems
Remote sensing