|
ACCESS THE FULL ARTICLE
No SPIE Account? Create one
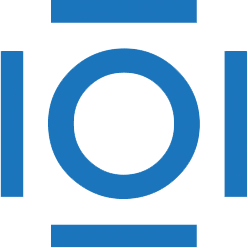
CITATIONS
Cited by 1 scholarly publication.
Feature selection
Particle swarm optimization
Genetic algorithms
Data acquisition
Particles
Stochastic processes
Hyperspectral imaging