|
ACCESS THE FULL ARTICLE
No SPIE Account? Create one
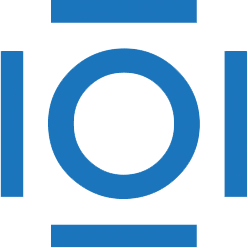
CITATIONS
Cited by 3 scholarly publications.
Sensors
Detection and tracking algorithms
Hyperspectral imaging
Target detection
Data modeling
Platinum
Statistical analysis