|
ACCESS THE FULL ARTICLE
No SPIE Account? Create one
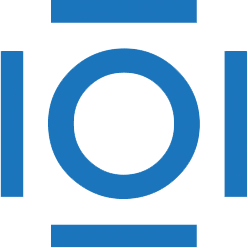
CITATIONS
Cited by 4 scholarly publications.
Detection and tracking algorithms
Forward looking infrared
Data modeling
Machine learning
Automatic target recognition
Binary data
Calibration