Nevertheless, by definition, PCA is meant to be applied to a single multispectral/hyperspectral image at a time. When several images have to be treated, running a PCA on each image would generate specific reduced spaces, which is not suitable for comparison between results. Thus, we focus on two PCA based algorithms that could define common reduced spaces of representation. The first method arises from literature and is computed with the barycenter covariance matrix. On the contrary, we designed the second algorithm with the idea of correcting standard PCA using permutations and inversions of eigenvectors. These dimensionality reduction methods are used within the context of a cosmetological study of a foundation make-up. Available data are in-vivo multispectral images of skin acquired on different volunteers in time series. The main purpose of this study is to characterize the make-up degradation especially in terms of texture analysis. Results have to be validate by statistical prediction of time since applying the product. PCA algorithms produce eigenimages that separately enhance skin components (pores, radiance, vessels...). From these eigenimages, we extract morphological texture descriptors and intent a time prediction. Accuracy of common reduced spaces outperform classical PCA one. In this paper, we detail how PCA is extended to the multiple groups case and explain what are the advantages of common reduced spaces when it comes to study several multispectral images. |
ACCESS THE FULL ARTICLE
No SPIE Account? Create one
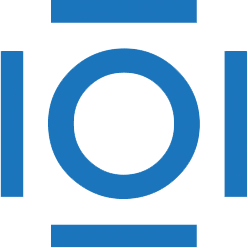
CITATIONS
Cited by 1 scholarly publication.
Principal component analysis
Multispectral imaging
Skin
Matrices
In vivo imaging
Promethium
Americium