|
ACCESS THE FULL ARTICLE
No SPIE Account? Create one
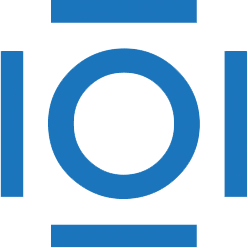
CITATIONS
Cited by 1 scholarly publication.
Image quality
Medical imaging
Tissues
Image processing
Image analysis
Machine learning
Denoising